Antibiotic resistance is a growing public health problem around the world. When bacteria like E. coli no longer respond to antibiotics, infections become harder to treat.
To develop new antibiotics, researchers typically identify the genes that make bacteria resistant. Through laboratory experiments, they observe how bacteria respond to different antibiotics and look for mutations in the genetic makeup of resistant strains that allow them to survive. While effective, this method can be time-consuming and may not always capture the full picture of how bacteria become resistant.
For example, changes in how genes work that don’t involve mutations can still influence resistance. Bacteria can also exchange resistance genes between each other, which may not be detected if only focusing on mutations within a single strain. My colleagues and I developed a new approach to identify E.
coli resistance genes by computer modeling, allowing us to design new compounds that can block these genes and make existing treatments more effective. Identifying resistance To predict which genes contribute to resistance, we analyzed the genomes of various E. coli strains to identify genetic patterns and markers associated with resistance.
We then used machine learning algorithms trained on existing data to highlight novel genes or mutations shared across resistant strains that might contribute to resistance. After identifying resistance genes, we designed inhibitors that specifically target and block.
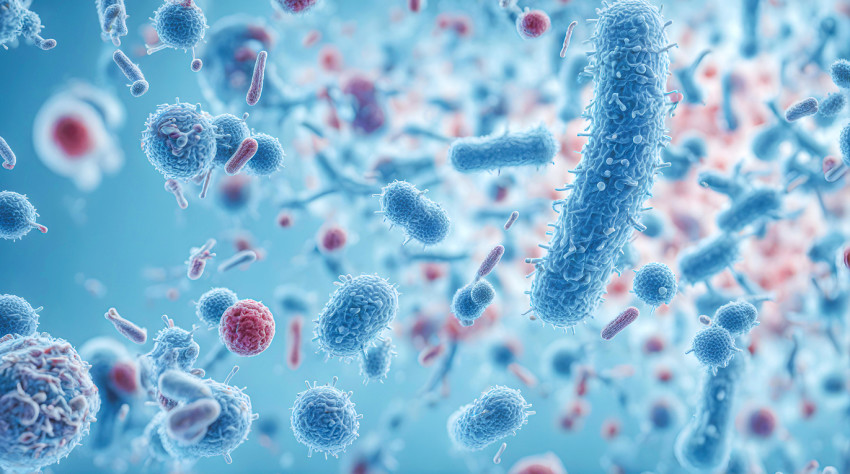