Artificial intelligence tools such as ChatGPT have been touted for their promise to alleviate clinician workload by triaging patients, taking medical histories and even providing preliminary diagnoses. These tools, known as large-language models, are already being used by patients to make sense of their symptoms and medical tests results. But while these AI models perform impressively on standardized medical tests, how well do they fare in situations that more closely mimic the real world? Not that great, according to the findings of a new study led by researchers at Harvard Medical School and Stanford University.
For their analysis, published Jan. 2 in Nature Medicine , the researchers designed an evaluation framework -; or a test -; called CRAFT-MD (Conversational Reasoning Assessment Framework for Testing in Medicine) and deployed it on four large-language models to see how well they performed in settings closely mimicking actual interactions with patients. All four large-language models did well on medical exam-style questions, but their performance worsened when engaged in conversations more closely mimicking real-world interactions.
This gap, the researchers said, underscores a two-fold need: First, to create more realistic evaluations that better gauge the fitness of clinical AI models for use in the real world and, second, to improve the ability of these tools to make diagnosis based on more realistic interactions before they are deployed in the clinic. Evaluation too.
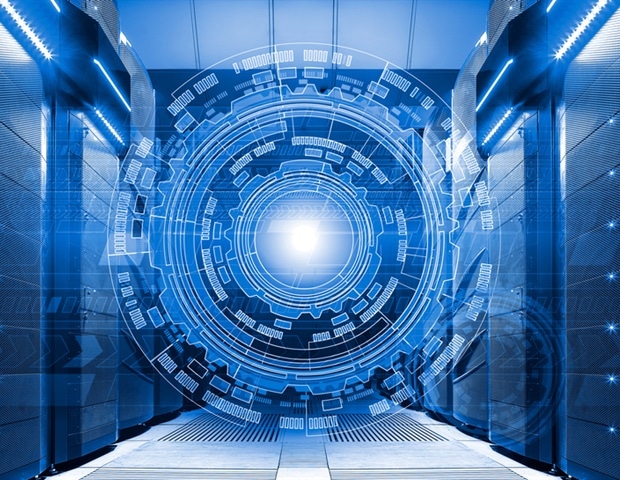